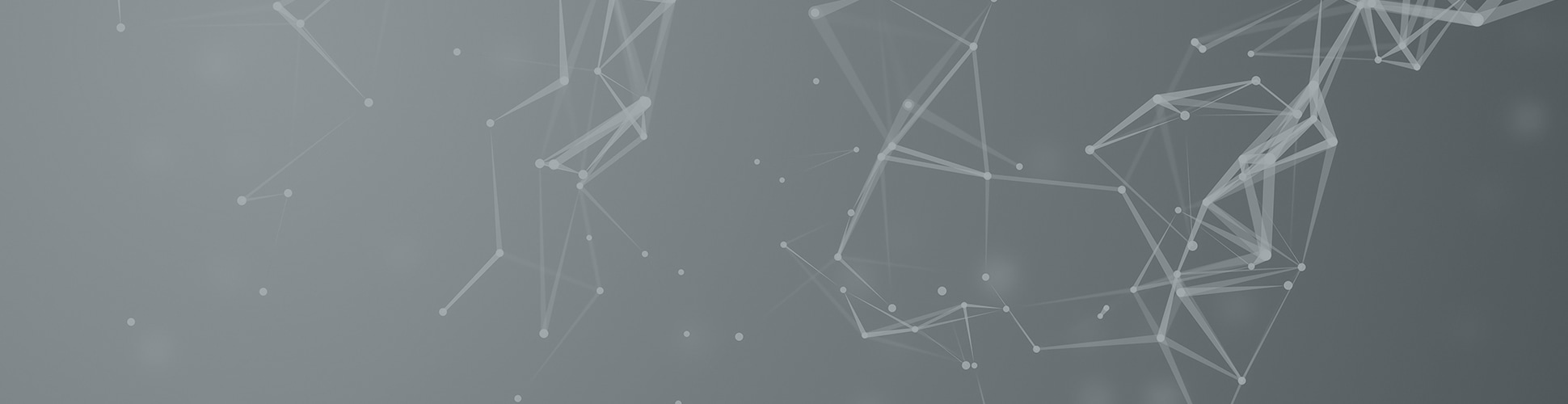
The Practical Applications of Modeling and Simulation
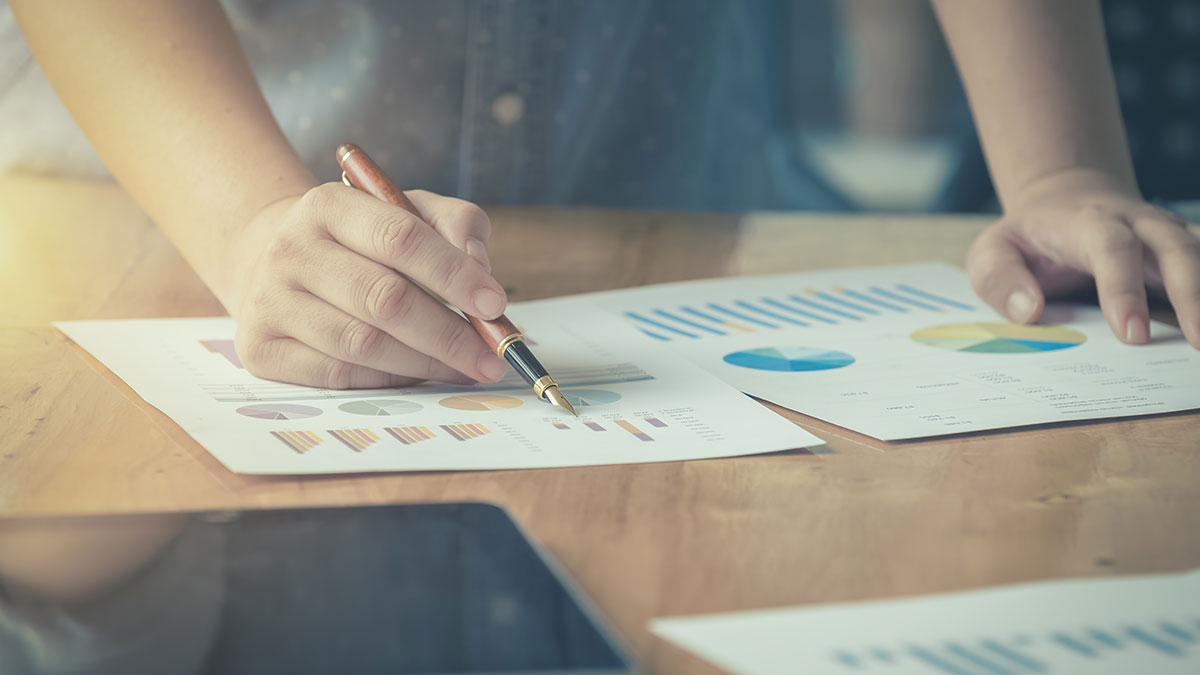
Steven Kathman, principal pharmacokineticist at PPD, discusses the importance of modeling and simulation in early development and how the data produced can enhance efficiencies in later phase trials.
The earlier in the drug development process that modeling starts, the better. Modeling preclinical data provides the first attempt at understanding or establishing what the dose, concentration and response relationships are. To demonstrate that an investigational product is safe and effective for the intended use, drug development programs commonly include multiple clinical trials. These programs generally begin with evaluating pharmacokinetics (PK), pharmacodynamics (PD), safety and tolerability in Phase I studies.
PPD provides comprehensive and flexible PK support for all phases of drug development. We offer preclinical pharmacology, clinical pharmacology and scientific expertise as part of an integrated drug development program in a variety of areas. Our pharmacokineticists are skilled and experienced in the analysis and interpretation of PK and PD data, providing assessment of clinical relevance and insight into results for use in drug development decisions.
What are PK and PD, and how do they relate to modeling and simulation?
PK, by definition, is the action of drugs in the body over a period of time, including the processes of absorption, distribution, localisation in tissues, biotransformation and excretion. In simple terms, what happens to the drug after it enters the body. This is assessed by measuring the concentration of the drug in blood (plasma or serum) over time. The data are often summarized through some metric such as area under the concentration time curve (AUC) or maximum observed concentration (Cmax).
PK modeling involves representing the concentration over time through a suitable mathematical function with dose(s) as an input and the concentration at various times as the output. The reasons for doing this are discussed below. These mathematical models are motivated by physiology and properties of the drug. How much physiological information gets incorporated depends in part on the amount of information available and what is needed to make accurate predictions.
PD, by definition, is the study of the biochemical and physiological effects of drugs and the mechanisms of their actions, including the correlation of actions and effects of drugs with their chemical structures. In simpler terms, it is the branch of pharmacology concerned with the effects of drugs and the mechanism of their action. This is also assessed by measuring some response to treatment over time, whether it be a biomarker (surrogate for response) or a clinical effect (e.g., survival time for oncology). The mathematical models depend on the mechanism of action for the drug and the physiology behind the PD outcome itself. How much of this gets incorporated into the model depends on the amount of information available and what is needed to make accurate predictions.
Like PK modeling, PD modeling involves representing the PD outcome through a suitable mathematical function. For PD modeling though, the input is some PK metric (concentration, AUC, Cmax) and the output is some PD measure or metric.
The PK and PD models are then often linked together in a PK/PD model, where the input is the dose(s) of the drug, which then leads to drug concentrations over time that are used to predict the PD measure or metric of interest.
Why is modeling and simulation important to drug development?
Physiologically motivated models that represent the PK and PD for a drug can be used to confirm hypothesized mechanisms of action. If the model represents the data well, then we have some confidence or support for the anticipated mechanisms of action. If the model does not represent the data well, then there is likely more happening than anticipated. This can then lead to further exploration to understand what is missing and build that into the model.
Once the model has been developed that represents the data well, then the model may be used to make predictions under various scenarios. This is often done early in drug development to assist with selecting doses and schedules to be explored in later trials. Primary reasons for drug failure in clinical trials are lack of efficacy or safety issues. It is important to ensure that the wrong dose or schedule is not the reason for this. Well-developed models can be used to perform simulations that assist with selecting the dose(s) and schedule(s) with the best chance of success.
Part of the model development process includes examining covariates (characteristics of the participants in an experiment) that may have an impact on the PK and/or PD. Covariates may include demographics (e.g., age, weight, gender), disease status (e.g., healthy volunteer, tumor type [oncology]), lab measures (kidney function, liver function), etc. The result of covariate analyses may include adjusting doses for subsets of patients or recommending treatment for only those with the best chance to truly benefit.
How can modeling and simulation of preclinical data be applied to first-in-human (FIH) study considerations and beyond?
The earlier in the drug development process that modeling starts, the better. Modeling preclinical data provides the first attempt at understanding or establishing what the dose/concentration/response relationships are. Once this is established, scaling may be performed to predict what the outcomes may be for human trials. If the model incorporates enough physiology, then scaling up may be done by accounting for the differences in physiology. For simpler models, some form of allometric scaling may be used that takes advantage of relationships in parameters versus species that have been established (though not always perfect) to some degree. The scaled up for human model can be used to perform simulations to inform study design of the FIH trial. Modeling and simulation are thus useful tools for establishing the starting dose for a FIH trial. This can be particularly useful in cases where the minimum anticipated biological effect level is required.
What is the correlation between toxicokinetic (TK) preclinical modeling with Phase I and higher clinical data?
The goal of TK modeling is to relate toxicity with levels of exposure. With proper consideration for differences in physiology, the results can be extrapolated to predict outcomes for humans. TK studies often require doses to be given to animals that yield higher exposures to induce the toxic effects. So, information from TK modeling can provide useful information for developing clinical (human) PK/PD models. This can assist with estimating some parameter values in the PK/PD model for humans that may otherwise be difficult to estimate due to data limitations.
How can you use modeling and simulation to enhance data generated in Phase II, III and IV?
Modeling can start early in the process and continue as more data are acquired. Modeling is a practical and efficient way to synthesize data and information across the entire drug development spectrum. While each study is conducted to obtain information and address specific questions, modeling the data from all studies together can address how the drug works (understanding the mechanisms of action), who the drug will most likely benefit (through examining covariates) and how best to deliver the drug. By using the model to synthesize the data in this way, decisions based on modeling are utilizing all of the information available at that time, not just the results of one study. Modeling can also help address issues such as what may happen if the drug is not used as intended (effects of missed doses or compliance issues in general); whether ethnic bridging studies are needed; and likely effects of renal or hepatic impairment (are studies for these likely needed).